| |
|
RMMJ
Rambam Maimonides Medical Journal
Rambam Health Care Campus 2018 January; 9(1): e0004.
ISSN: 2076-9172 Published online 2018 January 29. doi: 10.5041/RMMJ.10316
The Reliability of Surgical Apgar Score in Predicting Immediate and Late Postoperative Morbidity and Mortality: A Narrative Review Abhijit Nair, M.D.,* Aanchal Bharuka, D.A., D.N.B., and Basanth Kumar Rayani, D.A., D.N.B.
Department of Anesthesiology, Basavatarakam Indo-American Cancer Hospital and Research Institute, Hyderabad, Telangana, India
|
Surgical Apgar Score is a simple, 10-point scoring system in which a low score reliably identifies those patients at risk for adverse perioperative outcomes. Surgical techniques and anesthesia management should be directed in such a way that the Surgical Apgar Score remains higher to avoid postoperative morbidity and mortality.
Keywords: Apgar score, morbidity, mortality, postoperative complications, risk factors, surgery |
Surgical risk scoring is important to predict postoperative outcomes, to plan admission to the intensive care unit (ICU), to prognosticate the general condition of the surgical patient, and to plan specific interventions postoperatively. A unit which is not fully equipped with multispecialty areas could plan transfer of a patient to a more specialized center based on the risk score.
Virginia Apgar, an anesthesiologist, described the 10-point scoring system, the Apgar score, in 1952 for assessing newborn babies.1 Scoring is done at 1 min and 5 min after birth. The score is helpful in predicting overall outcome after resuscitation of a child. Anesthesiologists and surgeons anticipate the perioperative events involved after major surgeries (laparotomies, resection/anastomosis, vascular surgery, neurosurgeries, emergency or urgent surgery) on the basis of factors like age, associated co-morbidities, surgical blood loss, and surgery duration. An otherwise uneventful intraoperative course does not predict the postoperative course in any patient. Complications occurring after surgery, especially after patient discharge, lead to increased morbidity, increased cost of treatment in the form of hospital admissions, and unwanted interventions. |
The Physiological and Operative Severity Score for the Enumeration of Mortality and Morbidity (POSSUM), the Simplified Acute Physiology Score (SAPS), and Acute Physiology and Chronic Health Evaluation (APACHE) scoring systems have been used to predict postoperative course.
Copeland et al. initially described POSSUM in 1991, for predicting morbidity and mortality of surgical patients.2 In 1998, the Portsmouth modification or the P-POSSUM was described. The P-POSSUM was found to be more reliable and accurate when compared to the POSSUM described by Copeland.3 Twelve physiologic variables and six operative variables are used by POSSUM (Table 1). Although P-POSSUM also uses the same indices that are used for POSSUM, the equation used to calculate the score is different. All the values have to be entered, and the score is derived either by adding up or by using software. Moreover, many investigations such as hemoglobin, urea, white cell count, sodium, potassium, and electrocardiogram are required. Surgical events are also used for scoring (peritoneal soiling, multiple surgeries). There could be a lot of personal differences when certain entries are made like assessment of surgery and respiratory status. In addition, POSSUM is not applicable for trauma patients, and an overestimation of POSSUM is possible in hepatopancreatobiliary surgeries.4 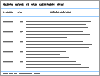 | Table 1 The 12 Physiologic Indices and Six Operative Indices Used for Calculating the POSSUM Score. |
Another score used to predict outcomes in medical and surgical patients is the Simplified Acute Physiology Score (SAPS). The SAPS II is used to score the status of patients admitted in the intensive care unit (ICU). It includes 17 variables: 12 physiologic variables, age, type of admission, and 3 disease-related variables. The SAPS II score registers the worst value of selected variables within the first 24 h after admission and can have a score between 0 and 163 points (0–116 points for physiologic variables, 0–17 points for age, and 0–30 points for previous diagnosis). Logistic regression is used to calculate the probability of death.5
The Acute Physiology and Chronic Health Evaluation (APACHE) II was developed using a database of North American ICU patients in 1985.6 It uses a score derived from 12 routine physiologic measurements taken during the first 24 h after admission, age, and previous medical issues to provide information about the severity of disease. A score from 0 to 71 is calculated based on these measurements. A higher score signifies a more severe disease with greater risk of death. The APACHE II has been used to prognosticate acutely ill patients and has helped researchers to compare the efficacy of various forms of treatment modalities. However, APACHE II led to an overestimation of mortality as physiologic variables used were dynamic and kept changing during treatment. Later APACHE III was introduced with two new variables: patient origin and the lead-time bias. Here, the score varied between 0 and 299 points.7 Later, APACHE IV was introduced in which another five variables were added: mechanical ventilation, thrombolysis, impact of sedation on Glasgow Coma Score (GCS), re-scaled GCS, and PaO2/FiO2 (arterial oxygen tension and fractional concentration of inspired oxygen) ratio.8
The SAPS and APACHE were found to be more reliable in predicting severity of condition and outcomes in medical patients when compared to surgical patients.9 Rapsang et al. have described nine routinely used scoring systems for predicting the morbidity and mortality of patients admitted in the ICU. The authors felt that selecting an inappropriate scoring methodology could lead to a significant waste of time, unwanted investigations, increased cost, and unwarranted extrapolations.10 Anesthesiologists use the American Society of Anesthesiologists–Physical Status (ASA-PS) classification for describing patients based on co-morbidities, functional status, and emergency or elective surgery. The ASA-PS was not designed to predict the mortality of a surgical patient. The ASA classification, with a positive predictive value of 57% for complications and a negative predictive value of 80%, is not considered reliable for predicting the 30-day postoperative course accurately.11 |
Gawande et al. described the Surgical Apgar Score (SAS) in 2007.12 The score was derived from a retrospective analysis of 303 patients who underwent colectomy at Brigham and Women’s Hospital, Boston, MA. This 10-point score is based on the patient’s surgical blood loss, the lowest intraoperative heart rate, and lowest recorded mean arterial pressure. The authors observed that as the score increased, outcomes improved at the end of 30 days. Many papers (discussed later on in this article) were subsequently published that interpreted prospective and retrospective data and concluded that SAS could accurately predict morbidity and complications in several surgical subspecialties. The SAS uses a 10-point scoring system that has been used to accurately predict early and 30-day postoperative complications in all major surgeries in the last decade. The 10-point SAS is shown in Table 2. 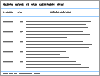 | Table 2 The 10-point Surgical Apgar Score. |
|
APPLICATION OF SAS BY OTHER RESEARCHERS IN OTHER SURGERIES
The correlation of SAS with perioperative morbidity and complications was different when used in different subspecialties by different researchers. However, when the patient’s ASA-PS classification was adjusted using relevant software, SAS scores remained associated with death and complications in several subspecialties. We searched PubMed, Scopus, Embase, and Google Scholar databases with the keywords “Surgical Apgar Score,” “Postoperative complications,” “Surgery,” “Morbidity,” and “Mortality” and identified 25 retrospective studies and 11 prospective studies that used SAS as a prognosticator tool to correlate with early and late postoperative complications (up to 30 days). The details of the retrospective and prospective data, type of surgical patients reviewed, total number of patients reviewed, and the reliability of SAS in predicting postoperative events are presented in Tables 3 and 4.13–49 Most of the published papers that have investigated the efficacy of SAS are based on retrospective data collected from electronic hospital records; the SAS was calculated from the records. The authors used univariate and multivariate analyses to assess factors associated with major postoperative complications. Data collected from the National Surgical Quality Improvement Program underwent logistic regression using 27 preoperative variables as predictors; the outcome was determined by using the incidence of major postoperative complications to generate a multivariable preoperative risk prediction model. 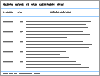 | Table 3 All Retrospective Studies Using SAS Scores for Various Surgeries to Predict Immediate and Delayed Postoperative Complications (30 days). |
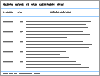 | Table 4 Prospective Studies Using SAS Scores for Various Surgeries to Predict Immediate and Delayed Postoperative Complications (30 days). |
Twenty out of 25 retrospective studies concluded that SAS correlated with adverse postoperative events. The SAS could not predict unfavorable events in patients who underwent knee arthroplasties (Wuerz et al.13), hysterectomy for malignancy (Clark et al.22), Ivor Lewis esophagectomies (Strøyer et al.19), spine surgery for metastasis (Lau et al.25), gastrectomy (Miki et al.21), and microvascular head and neck reconstruction (Ettinger et al.35). The authors felt that preoperative functional status and age were stronger predictors than SAS. Out of the 11 prospective studies, one study that analyzed patients undergoing orthopedic surgeries suggested that SAS could not predict adverse surgical outcomes. Haddow et al.39 analyzed 143 general and vascular surgical patients, and suggested conducting a randomized control trial due to the few cases, which provided inconclusive data. Thorn et al.47 found that general and vascular surgery patients with a lower SAS correlated well with postoperative outcomes. However, there was poor correlation in orthopedic patients. The initial hypotension that occurs after administering a spinal anesthetic may explain the poor SAS correlation of postoperative events in these patients.
Hypotension usually improves with crystalloid boluses or a few doses of vasopressors. House et al.50 retrospectively analyzed data from 2007–2012 and found that a low SAS score was due to increased cardiac troponin levels after non-cardiac surgery. Out of 46,799 patients, 209 (0.4%) had increased troponins and 192 (0.4%) patients experienced myocardial infarction following non-cardiac surgeries.50 Jering et al.51 used the Area Under the Receiver Operating Characteristic Curve (AUROC) and suggested that combining ASA physical classification with continuously measured SAS was better in predicting major postoperative complications than using ASA physical status and SAS alone.51 |
An ideal surgical risk scoring system should be simple; require minimal calculation, data and variables; be reasonably accurate; and must be objective, economical, and suitable for all situations (elective/emergency surgeries and valid in all specialties). The SAS is a simple way to predict complications during the postoperative period. It is a simple and inexpensive scoring system that can reliably predict serious postoperative consequences relying on only three variables. Patients undergoing major thoracic, abdominal, and vascular surgeries with significant co-morbidities are expected to have adverse perioperative outcomes. In spite of this, the SAS was able to predict either alone, or in combination with associated risk factors, the occurrence of life-threatening events in the postoperative period. A patient with a low intraoperative SAS should be considered at risk and monitored meticulously. A patient with a low SAS should be monitored for an extended period in the ICU.
The SAS does not appear to correlate well with surgeries performed under regional anesthesia (e.g. arthroplasties, as shown by Wuerz et al.13 and Thorn et al.47). Well-designed prospective studies in the future could provide better insight into the reason behind the lack of correlation between regional anesthesia and SAS. The score could also help surgeons to improve or change their practice. This might include, for example, preventing surgical bleeds by meticulous use of electrocautery, identifying and ligating possible bleeders, and/or using a tourniquet whenever possible; giving time for bleeding to maintain mean arterial pressure (MAP); and avoiding events that lead to severe bradycardia by using slow insufflations after port insertion, maintaining normal intra-abdominal pressure, and avoiding forceful omental/peritoneal handling.
Hyder et al.52 investigated the effect on the SAS of different sampling methods for extracting vital signs data. In the study that involved more than 3,000 patients, they found that larger SAS sampling intervals resulted in better model discrimination and improved reclassification. The authors had a large sample size, studied a variety of non-cardiac surgeries, and had a detailed classification of preoperative and postoperative morbidity. Optimized algorithms and larger sampling intervals of required parameters are needed to use SAS to predict patients at risk for adverse postoperative events. Smaller sampling intervals could lead to inadequate data, leading the investigator to find SAS unsatisfactory in predicting adverse outcomes. |
The SAS is a simple 10-point scoring system that can be easily calculated and entered in case records at the end of surgery. Unlike other scoring systems, SAS does not require biochemical investigations, clinical assessment, acute or chronic disease classification, or depend on the timing of the surgery (elective, urgent, emergency). The patient with a low SAS could experience adverse perioperative life-threatening events during the first 30 days of the postoperative period. Using SAS helps to identify patients at risk, and contributes to post-procedural auditing with evidence-based methodologies to help achieve the highest possible SAS in a surgical patient. In addition, SAS along with ASA-PS and larger sampling intervals could help to identify patients in need of monitoring and vigilant follow-up during the postoperative period. |
APACHE |
Acute Physiology and Chronic Health Evaluation |
ASA-PS |
American Society of Anesthesiologists–Physical Status |
GCS |
Glasgow Coma Score |
ICU |
intensive care unit |
POSSUM |
Physiological and Operative Severity Score for the Enumeration of Mortality and Morbidity |
P-POSSUM |
Portsmouth modification POSSUM |
SAPS |
Simplified Acute Physiology Score |
SAS |
Surgical Apgar Score |
|
|
4. Wang H, Chen T, Wang H, Song Y, Li X, Wang J. A systematic review of the Physiological and Operative Severity Score for the enumeration of Mortality and morbidity and its Portsmouth modification as predictors of post-operative morbidity and mortality in patients undergoing pancreatic surgery. Am J Surg. 2013;205:466–72. https://doi.org/10.1016/j.amjsurg.2012.06.011. 7. Knaus WA, Wagner DP, Draper EA, et al. The APACHE III prognostic system. Risk prediction of hospital mortality for critically ill hospitalized adults. Chest. 1991;100:1619–36. https://doi.org/10.1378/chest.100.6.1619. 8. Zimmerman JE, Kramer AA, McNair DS, Malila FM, Shaffer VL. Intensive care unit length of stay: benchmarking based on Acute Physiology and Chronic Health Evaluation (APACHE) IV. Crit Care Med. 2006;34:2517–29. https://doi.org/10.1097/01.CCM.0000240233.01711.D9. 9. Gilani MT, Razavi M, Azad AM. A comparison of Simplified Acute Physiology Score II, Acute Physiology and Chronic Health Evaluation II and Acute Physiology and Chronic Health Evaluation III scoring system in predicting mortality and length of stay at surgical intensive care unit. Niger Med J. 2014;55:144–7. https://doi.org/10.4103/0300-1652.129651. 13. Wuerz TH, Regenbogen SE, Ehrenfeld JM, Malchau H, Rubash HE, Gawande AA. The Surgical Apgar Score in hip and knee arthroplasty. Clin Orthop Relat Res. 2011;469:1119–26. https://doi.org/10.1007/s11999-010-1721-x. 14. Regenbogen SE, Bordeianou L, Hutter MM, Gawande AA. The intraoperative Surgical Apgar Score predicts post-discharge complications after colon and rectal resection. Surgery. 2010;148:559–66. https://doi.org/10.1016/j.surg.2010.01.015. 16. Sobol JB, Gershengornayley B, Wunsch H, Li G. The Surgical Apgar Score is strongly associated with ICU admission after high-risk intra-abdominal surgery. Anesth Analg. 2013;117:438–46. https://doi.org/10.1213/ANE.0b013e31829180b7. 17. Xing XZ, Wang HJ, Qu SN, et al. The value of esophagectomy Surgical Apgar Score (eSAS) in predicting the risk of major morbidity after open esophagectomy. J Thorac Dis. 2016;8:1780–7. https://doi.org/10.21037/jtd.2016.06.28. 19. Strøyer S, Mantoni T, Svendsen LB. Evaluation of the Surgical Apgar Score in patients undergoing Ivor-Lewis esophagectomy. J Surg Oncol. 2017;115:186–91. https://doi.org/10.1002/jso.24483. 20. Eto K, Yoshida N, Iwatsuki M, et al. Surgical Apgar Score predicted postoperative morbidity after esophagectomy for esophageal cancer. World J Surg. 2016;40:1145–51. https://doi.org/10.1007/s00268-016-3425-1. 21. Miki Y, Tokunaga M, Tanizawa Y, Bando E, Kawamura T, Terashima M. Perioperative risk assessment for gastrectomy by Surgical Apgar Score. Ann Surg Oncol. 2014;21:2601–7. https://doi.org/10.1245/s10434-014-3653-2. 22. Clark RM, Lee MS, Alejandro Rauh-Hain J, et al. Surgical Apgar Score and prediction of morbidity in women undergoing hysterectomy for malignancy. Gynecol Oncol. 2015;136:516–20. https://doi.org/10.1016/j.ygyno.2014.11.016. 23. Assifi MM, Lindenmeyer J, Leiby BE, et al. Surgical Apgar Score predicts perioperative morbidity in patients undergoing pancreaticoduodenectomy at a high-volume center. J Gastrointest Surg. 2012;16:275–81. https://doi.org/10.1007/s11605-011-1733-1. 25. Lau D, Yee TJ, La Marca F, Patel R, Park P. Utility of the Surgical Apgar Score for patients who undergo surgery for spinal metastasis. Clin Spine Surg. 2016 Jun 28; [Epub ahead of print]. 26. Wied C, Foss NB, Kristensen MT, Holm G, Kallemose T, Troelsen A. Surgical Apgar Score predicts early complication in transfemoral amputees: retrospective study of 170 major amputations. World J Orthop. 2016;7:832–8. https://doi.org/10.5312/wjo.v7.i12.832. 27. Reynolds PQ, Sanders NW, Schildcrout JS, Mercaldo ND, St Jacques PJ. Expansion of the Surgical Apgar Score across all surgical subspecialties as a means to predict postoperative mortality. Anesthesiology. 2011;114:1305–12. https://doi.org/10.1097/ALN.0b013e318219d734. 29. Aoyama T, Kazama K, Murakawa M, et al. The Surgical Apgar Score is an independent prognostic factor in patients with pancreatic cancer undergoing pancreatoduodenectomy followed by adjuvant chemotherapy. Anticancer Res. 2016;36:2497–503. 30. Orberger M, Palisaar J, Roghmann F, et al. Association between the Surgical Apgar Score and perioperative complications after radical prostatectomy. Urol Int. 2017;98:61–70. https://doi.org/10.1159/000450795. 32. Yamada T, Tsuburaya A, Hayashi T, et al. Usefulness of Surgical Apgar Score on predicting survival after surgery for gastric cancer. Ann Surg Oncol. 2016;23(Suppl 5):757–63. https://doi.org/10.1245/s10434-016-5525-4. 33. Masi A, Amodeo S, Hatzaras I, et al. Use of the Surgical Apgar Score to enhance Veterans Affairs Surgical Quality Improvement Program surgical risk assessment in veterans undergoing major intra-abdominal surgery. Am J Surg. 2017;213:696–705. https://doi.org/10.1016/j.amjsurg.2016.05.017. 34. Stoll WD, Taber DJ, Palesch SJ, Hebbar L. Utility of the Surgical Apgar Score in kidney transplantation: is it feasible to predict ICU admission, hospital readmission, length of stay, and cost in this patient population? Prog Transplant. 2016;26:122–8. https://doi.org/10.1177/1526924816640948. 35. Ettinger KS, Moore EJ, Lohse CM, Reiland MD, Yetzer JG, Arce K. Application of the Surgical Apgar Score to microvascular head and neck reconstruction. J Oral Maxillofac Surg. 2016;74:1668–77. https://doi.org/10.1016/j.joms.2016.02.013. 36. Sakan S, Pavlovic DB, Milosevic M, Virag I, Martinovic P, Dobric I. Implementing the Surgical Apgar Score in patients with trauma hip fracture. Injury. 2015;46(Suppl 6):S61–6. https://doi.org/10.1016/j.injury.2015.10.051. 37. La Torre M, Ramacciato G, Nigri G, et al. Post-operative morbidity and mortality in pancreatic surgery. The role of Surgical Apgar Score. Pancreatology. 2013;13:175–9. https://doi.org/10.1016/j.pan.2013.01.011. 38. Ejaz A, Gani F, Frank SM, Pawlik TM. Improvement of the Surgical Apgar Score by addition of intraoperative blood transfusion among patients undergoing major gastrointestinal surgery. J Gastrointest Surg. 2016;20:1752–9. https://doi.org/10.1007/s11605-016-3234-8. 41. Urrutia J, Valdes M, Zamora T, Canessa V, Briceno J. Can the Surgical Apgar Score predict morbidity and mortality in general orthopaedic surgery? Int Orthop. 2012;36:2571–6. https://doi.org/10.1007/s00264-012-1696-1. 42. Prasad SM, Ferreria M, Berry AM, et al. Surgical Apgar outcome score: perioperative risk assessment for radical cystectomy. J Urol. 2009;181:1046–52. discussion 1052–3. https://doi.org/10.1016/j.juro.2008.10.165. 44. Ngarambe C, Smart BJ, Nagarajan N, Rickard J. Validation of the Surgical Apgar Score after laparotomy at a tertiary referral hospital in Rwanda. World J Surg. 2017;41:1734–42. https://doi.org/10.1007/s00268-017-3951-5. 45. Haynes AB, Regenbogen SE, Weiser G, et al. Surgical outcome measurement for a global patient population: validation of the Surgical Apgar Score in 8 countries. Surgery. 2011;149:519–24. https://doi.org/10.1016/j.surg.2010.10.019. 48. Ito T, Abbosh PH, Mehrazin R, et al. Surgical Apgar Score predicts an increased risk of major complications and death after renal mass excision. J Urol. 2015;193:1918–22. https://doi.org/10.1016/j.juro.2014.11.085. 49. Cihoric M, Toft Tengberg L, Bay-Nielsen M, Bang Foss N. Prediction of outcome after emergency high-risk. intra-abdominal surgery using the Surgical Apgar Score. Anesth Analg. 2016;123:1516–21. https://doi.org/10.1213/ANE.0000000000001501. 51. Jering MZ, Marolen KN, Shotwell MS, Denton JN, Sandberg WS, Ehrenfeld JM. Combining the ASA physical classification system and continuous intraoperative Surgical Apgar Score measurement in predicting postoperative risk. J Med Syst. 2015;39:147. https://doi.org/10.1007/s10916-015-0332-1. 52. Hyder JA, Kor DJ, Cima RR, Subramanian A. How to improve the performance of intraoperative risk models: an example with vital signs using the Surgical Apgar Score. Anesth Analg. 2013;117:1338–46. https://doi.org/10.1213/ANE.0b013e3182a46d6d. |